Machine Learning-Based Automated Detection of Seafloor Gas Seeps
Project Summary
Recognizing the importance of seeps and the significant potential for further seep discovery, a research team has been developing an efficient and cost-effective machine learning-based software system to automatically detect seafloor gas seeps (i.e., cold seeps) in mapping sonar water column data. Seeps are hotspots of deep-sea biodiversity, an important part of the global carbon cycle, indicators of potential marine geohazards, and an energy production resource. However, the deep ocean is largely unexplored, and most seeps are discovered through manual visual analysis of water column sonar imagery, which is time consuming, costly, and inconsistent. This project is designed to automate that process, accelerating the speed, accuracy, and consistency of seep discovery, and resulting in improved understanding of seep abundance and distribution.
Training the Model
The core element of the new system is a machine learning model, which needed to be trained to detect seeps. To train and validate the model, the team relied on multibeam sonar water column data collected by NOAA Ocean Exploration during expeditions along the U.S. Atlantic margin on NOAA Ship Okeanos Explorer. They visually reviewed over 428,396 multibeam sonar water column images — searching for gas bubble plumes — and digitally labeled them, noting the presence or absence of seep targets. Seep targets were present in 6,583 (1.54%) of these images. Where seeps were present, the researchers drew bounding boxes around the seep targets to indicate their locations, which resulted in 8,324 bounding boxes.
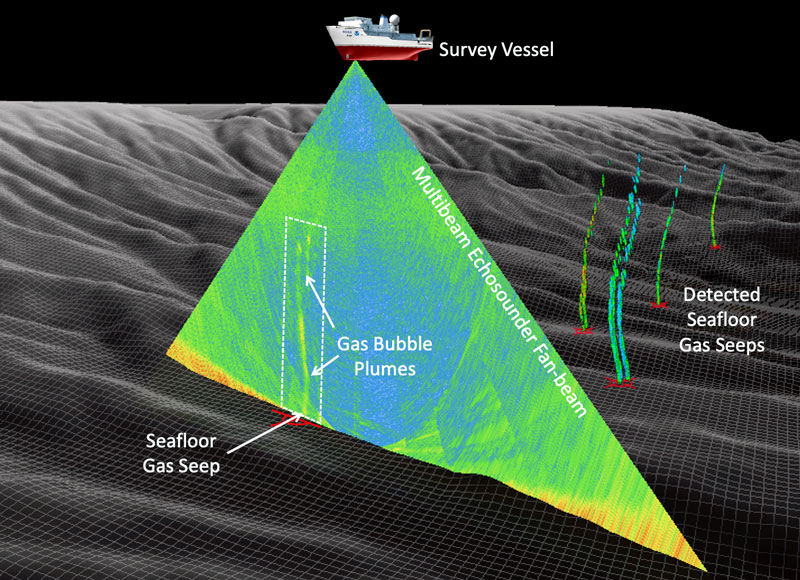
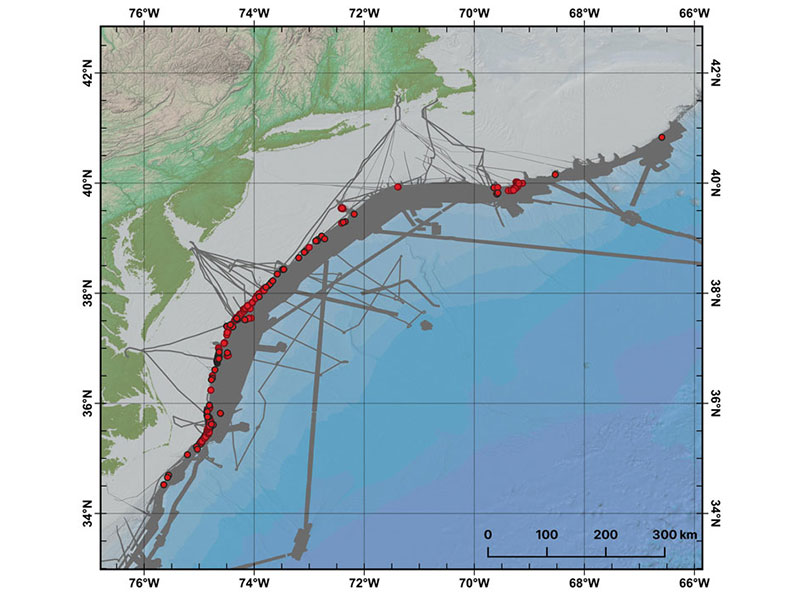
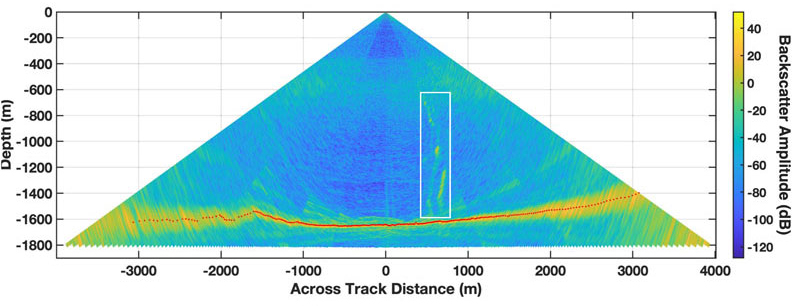
Next Steps
Next steps for this project include applying the approach to multibeam sonar data at the National Centers for Environmental Information that have not yet been reviewed for the presence of seeps, developing algorithms to estimate seafloor seep origin (latitude, longitude, and depth) for each gas plume the model detects, and continuing refinements. Additionally, the team is working toward using the system while multibeam sonar data are being collected to detect seeps in near real time. This will give researchers the ability to rapidly recognize and potentially respond to the presence of gas seeps while the survey ship is at sea.
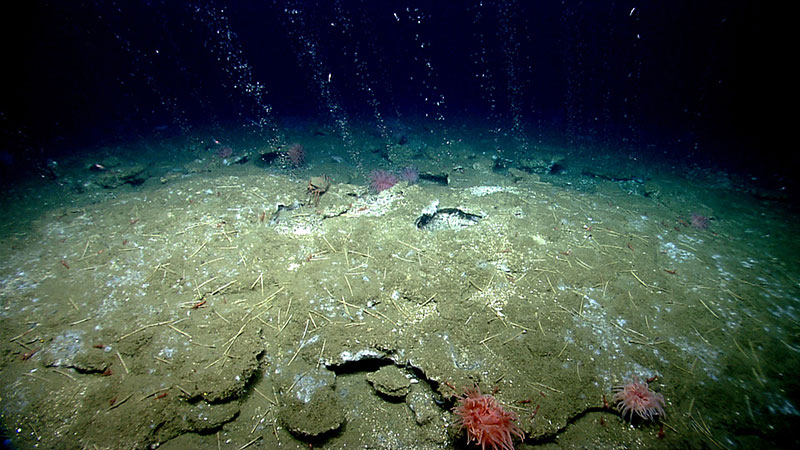
Expected Outcomes
The primary outcome of this project is a new technology that is expected to increase the speed, accuracy, and consistency of seep detection, while also decreasing costs and personnel requirements. Another anticipated outcome is the discovery of new seeps. Together, these and other outcomes will improve our basic understanding of the quantity and location of seafloor gas seeps around the world, providing critical deep-ocean data necessary for effective management and protection of ocean resources.
Meet the Explorers
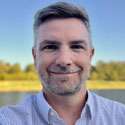
Adam Skarke
Principal Investigator
Mississippi State University
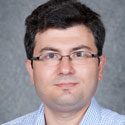
Ali C. Gurbuz
Co-Principal Investigator
Mississippi State University
Education Content
Education Theme pages provide the best of what the NOAA Ocean Exploration website has to offer to support your classroom during this expedition. On each theme page, you will find links to expedition features, lessons, multimedia, career information, and associated past expeditions.
Related Links
- What is the difference between a cold seep and a hydrothermal vent?
- Understanding of Methane Seep Formation and Prevalence on U.S. Atlantic Margin Advanced by New Study
- Methane Bubbling Up From the Atlantic Seafloor. Who Knew?
- The 100th NOAA Ship Okeanos Explorer Mission Visits New Methane Plumes Where the U.S. Atlantic Seeps Story Began
- An Update on Cold Seeps in the Northwestern Atlantic Ocean
- Discoveries at a Methane Seep Field Offshore Bodie Island, North Carolina
- Methane Seeps Discovered Along U.S. Atlantic Seafloor Using Data from Okeanos Explorer Expeditions
- Exploration of Cold Seeps on the North Atlantic Continental Margin
- Multibeam Sonar
Partners
- Mississippi State University Department of Geosciences
- Mississippi State University Department of Electrical and Computer Engineering
Media Contact
Emily Crum
Communications Specialist
NOAA Ocean Exploration
ocean-explore-comms@noaa.gov
Funding for this project was provided by NOAA Ocean Exploration via its Ocean Exploration Fiscal Year 2022 Funding Opportunity.
Published October 30, 2024